Research
On-Going Projects
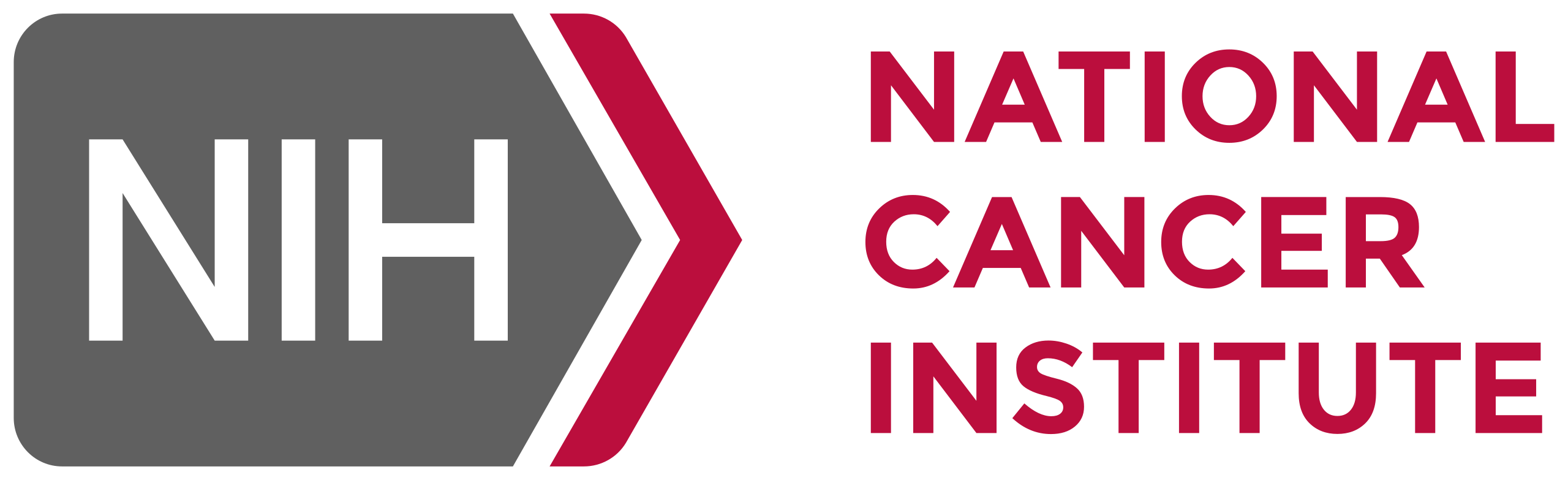
Improving ECP-CANDLE’s drug response prediction models: Bioinformatics and Machine Learning...
...
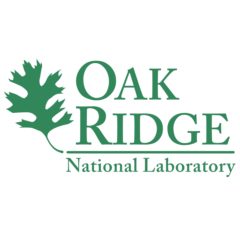
Exploring programming model and workflow for PiConGPU, in collaboration with ORNL and HZDR Germany...
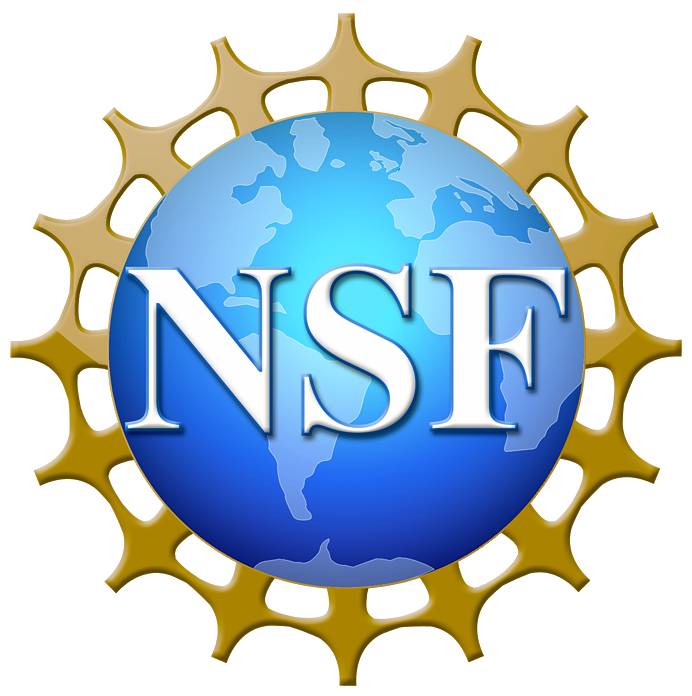
A compiler infrastructure that facilitates research on programming abstractions/models and compiler optimizations/constructions...
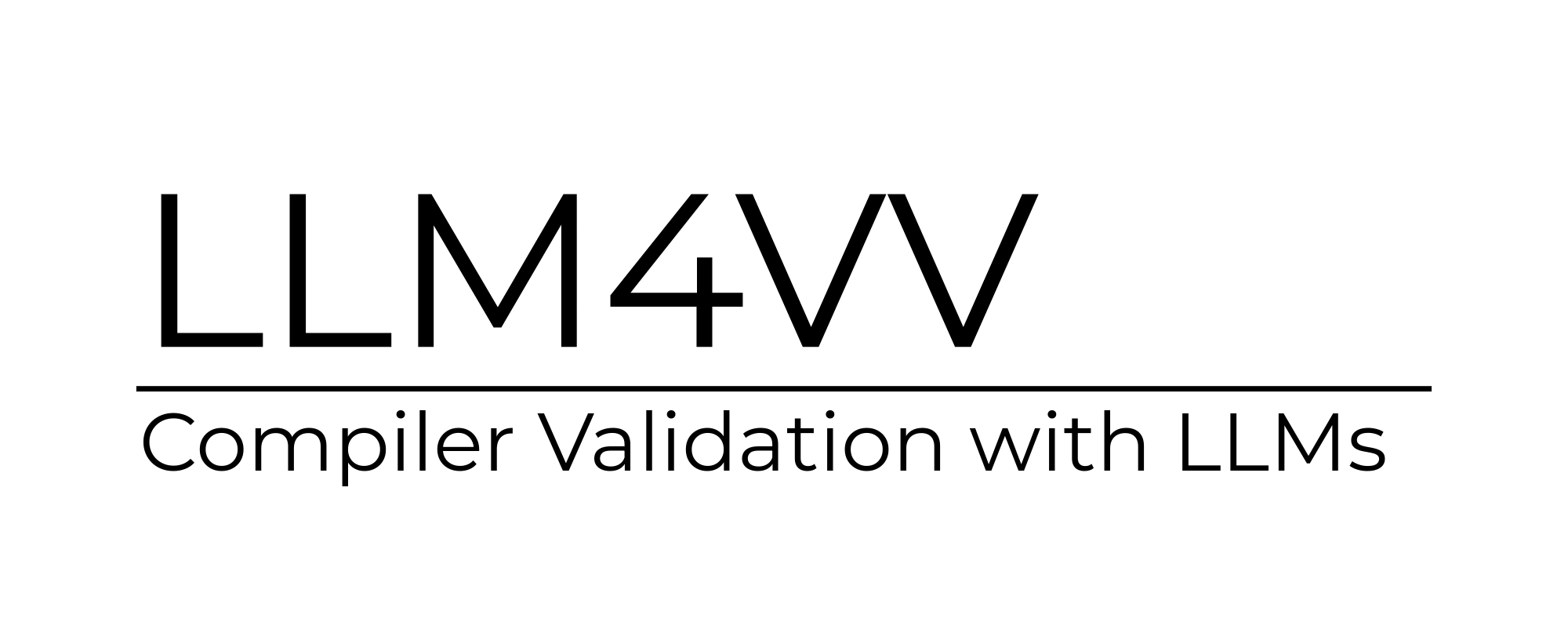
LLM4VV: Developing LLM-Driven Testsuite for Compiler Validation...
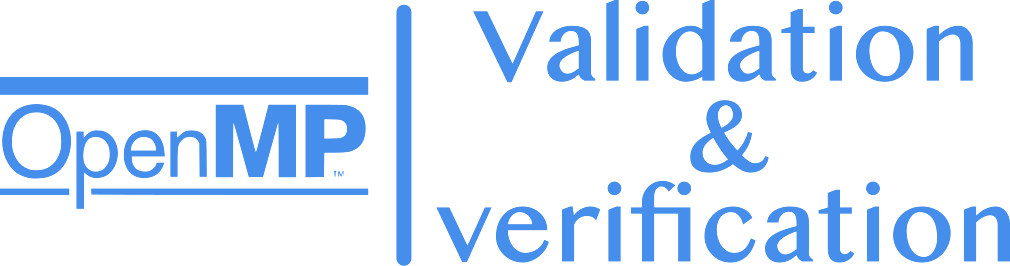
Building validation & verification (V&V) suites to validate the implementations of OpenMP 4.5+ offloading features...
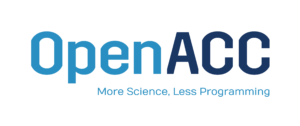
Building an OpenACC Validation & Verification (V&V) testsuite to validate implementations of OpenACC 2.5 features...
Completed Projects
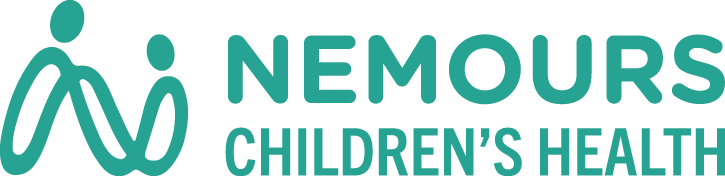
Building predictive models for rare disease outcomes using machine learning approaches and multiomic data...
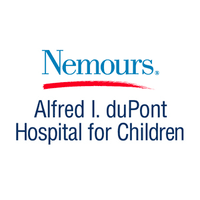
Building a portable and fast DNA sequence alignment tool...
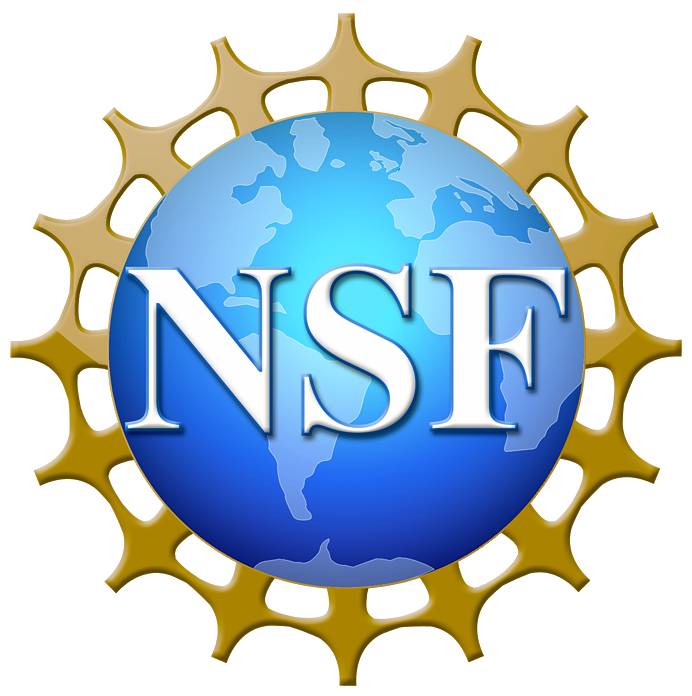
Building accelerator benchmark suite to stress test hardware, in collaboration with Standard Performance Evaluation Corporation High Performance Group (SPEC HPG)...
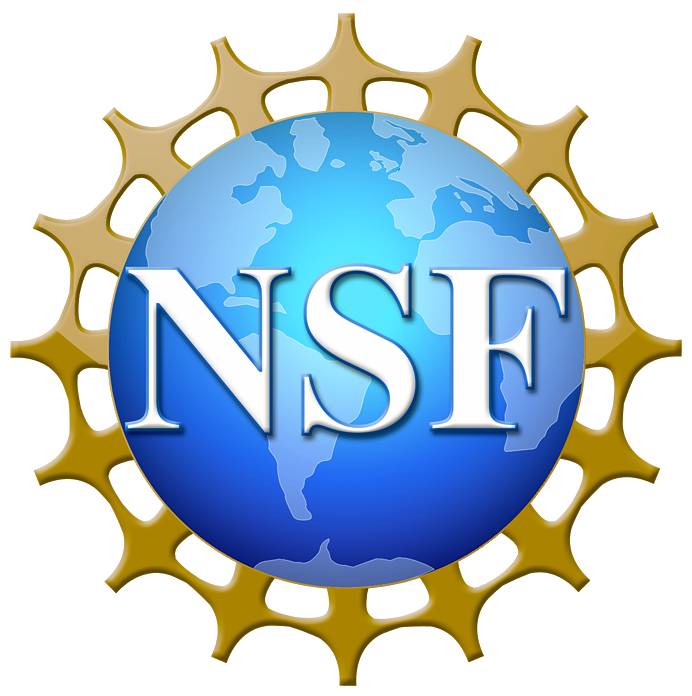
Exploring programming language features applicable to real-world applications...
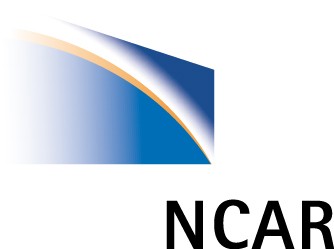
Acceleration of the MURaM Solar Physics Model using GPUs and OpenACC...
Github Repositories
In IEEE Proceedings of third Workshop on Accelerator Programming Using Directives (WACCPD), pp. 79-88, Salt Lake City, UT, November 2016....
Development of an accelerated version of the prediction of chemical shift of protein structures on GPUs using OpenACC on GPUs. This is the first directive-based version of the software that is available....
To appear in Proceedings of the Seventh International Workshop on Accelerators and Hybrid Exascale Systems (AsHES). IEEE Press, 2017....
This repository contains software developed using a portable, high-level framework using a popular MapReduce framework, Apache Spark, in conjunction with CUDA and OpenCL to take advantage of automatic data distribution and specialized hardware distributed across systems...
Undergraduate research project conducted within the Vertically Integrated Projects (VIP) program at the University of Delaware. Won 1st place at the VIP Mid-Atlantic poster competition, 2018....
In this code, we carefully utilize OpenACC, adirective-based programming model to accelerate the diffusion portion in Equation 1 inPhysiCell, a cross-platform agent-based biosimulationframework that has been adopted in cancer infectious diseases and othercomplex biological problem....
Work in Collaboration with Oak Ridge National Lab. Accelerating DOE’s Minisweep miniapp on supercomputers. Accepted, To be published in ACM Proceedings of The Platform for Advanced Scientific Computing (PASC) 2018....